Crypto x AI: 10 Categories We're Watching in 2025
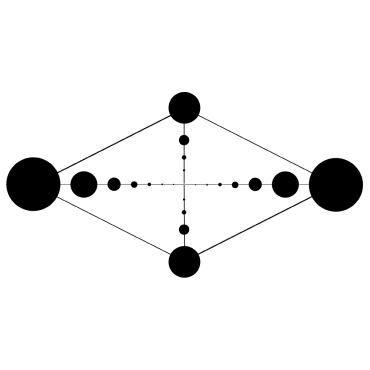
1. Agent-to-Agent Interaction
The default transparency and composability of blockchains make them a perfect substrate for agent-to-agent interaction, where agents developed by different entities for different purposes can interact with each other seamlessly. There’s already been great experimentation of agents sending funds to each other, launching tokens together, and more. We’d love to see how agent-to-agent interaction can scale both by creating net-new application spaces, such as new social venues driven by agent interactions, as well as by improving enterprise workflows we know to be tedious today, from platform authentication and verification to micropayments, inter-platform workflow integrations, and more.
- Danny, Katie, Aadharsh, Dmitriy

2. Decentralized Agentic Organizations
Multi-agent coordination at-scale is a similarly exciting area of research. How can multi-agent systems work together to complete tasks, solve problems, and govern systems and protocols? In his post at the beginning of 2024, “The promise and challenges of crypto + AI applications,” Vitalik references utilizing AI agents for prediction markets and adjudication. At scale, he essentially posits, multi-agent systems have remarkable capability for “truth”-finding and generally autonomous governance systems. We’re interested to see how the capabilities of multi-agent systems and forms of “swarm intelligence” continue to be discovered and experimented with.
As an extension of agent-to-agent coordination, agent-to-human coordination is an interesting design space––specifically how communities engage around agents or how agents organize humans to do collective action. We would love to see more experimentation with agents whose objective function involves large-scale human coordination. This will need to be paired with some verification mechanism, especially if the human work was done offchain, but there could be some very strange and interesting emergent behavior.
3. Agentic Multimedia Entertainment
The concept of digital personas has existed for decades. Hatsune Miku (2007) has sold out 20,000-seat venues and Lil Miquela (2016) has 2M+ followers on Instagram. Newer, lesser-known examples include the AI vtuber Neuro-sama (2022) who has 600K+ subscribers on Twitch and the pseudonymous kpop boy group PLAVE (2023), which has amassed over 300M+ views on YouTube in less than two years. With advancements in AI infrastructure and the integration of blockchains for payments, value transfer, and open data platforms, we’re excited to see how these agents can become more autonomous and potentially unlock a new mainstream category of entertainment in 2025.
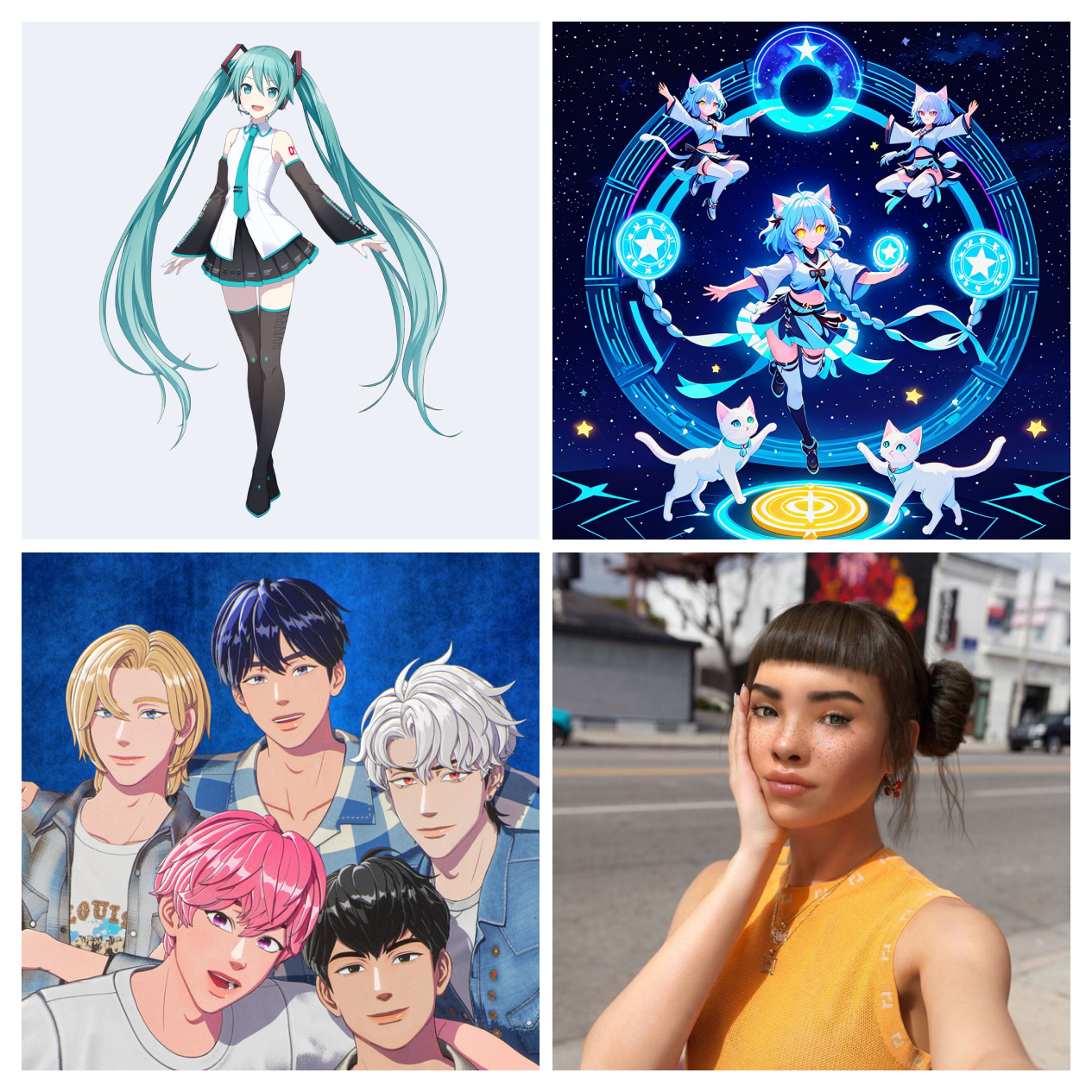
4. Generative/Agentic Content Marketing
Where in the previous case, the agent *is* the product, there’s also the case where agents can supplement products. In an attention economy, sustaining a constant stream of compelling content is crucial for the success of any idea, product, company, etc. Generative/agentic content is a powerful tool teams can use to ensure a scalable, 24/7 content origination pipeline. This idea space has been accelerated by the discussion around what distinguishes a memecoin from an agent. Agents are a powerful way for memecoins to gain distribution, even if not strictly “agentic” (yet).
As another example, games are increasingly expected to be more dynamic to maintain users’ engagement. One classic method for creating dynamism in games is to cultivate user-generated content; purely generative content (from in-game items, to NPCs, to entirely generative levels) is perhaps the next era to this evolution. We’re curious to see how far the boundaries of traditional distribution strategy will be extended by agent capabilities in 2025.
- Katie
5. Next-Gen Art Tools/Platforms
In 2024, we launched IN CONVERSATION WITH, an interview series with artists in/at the edges of crypto across music, visual art, design, curation, and more. One of the key observations I took from this year’s interviews was that artists interested in crypto are often interested in frontier technology more broadly, as well as having that technology live more squarely within the aesthetics or focus of their practice, i.e. AR/VR objects, code-based art, livecoding.
Generative art in particular has historically seen synergies with blockchains, making its potential as a similar substrate for AI art more clear. Showcasing and displaying these mediums of art properly is incredibly difficult to achieve in traditional forums. ArtBlocks provided a glimpse for what the future of digital art presentation, storage, monetization, and preservation leveraging blockchains could look like—improving the overall experience for both artists and viewers. Beyond presentation, AI tools have even extended the capability of laypeople to create their own art. It will be very interesting to see how blockchains can extend or power these tools in 2025.
- Katie
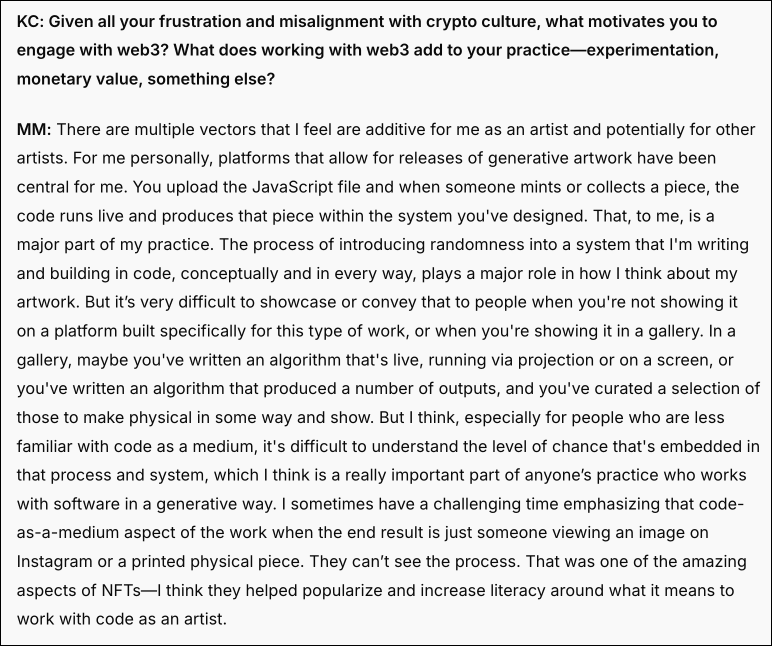
6. Data Markets
In the 20 years since Clive Humby coined the phrase “data is the new oil,” companies have taken robust measures to hoard and monetize user data. Users have woken up to the reality that their data is the foundation upon which these multi-billion dollar companies are built, yet they have very little control over how their data is leveraged nor exposure to the profit that it helps generate. The acceleration of powerful AI models makes this tension even more existential. If combating user exploitation is one part of the data opportunity, the other concerns solving for data supply shortfalls as ever larger and better models drain the easily accessible oilfields of public internet data and demand new sources.
On the first question of how we can use decentralized infrastructure to transfer the power of data back to its point of origin (users), it's a vast design space that requires novel solutions across a range of areas. Some of the most pressing include: where data is stored and how we preserve privacy (during storage, transfer and compute), how we objectively benchmark, filter, and value data quality, what mechanisms we use for attribution and monetization (especially when tying value back to source post-inference), and what orchestration or data retrieval systems we use in a diverse model ecosystem.
On the second question of solving supply constraints, it's not just about trying to replicate Scale AI with tokens, but understanding where we can have an edge given technical tailwinds and building thoughtful solutions with a competitive advantage, be it around scale, quality, or better incentive (and filtering) mechanisms to originate a higher value data product. Especially as much of the demand side continues to come from web2 AI, thinking through how we bridge smart contract-enforced mechanisms with conventional SLAs and instruments is an important area to be aware of.
- Danny

7. Decentralized Compute
If data is one fundamental building block in the development and deployment of AI, compute is the other. The legacy paradigm of large data centers with unique access to sites, energy, and hardware has largely defined the trajectory of deep learning and AI over the last few years, but physical constraints alongside open-source developments are starting to challenge this dynamic.
v1 of compute in decentralized AI looked like a replica of web2 GPU clouds with no real edge on supply (hardware or data centers) and minimal organic demand. In v2, we're beginning to see some remarkable teams build proper tech stacks over heterogeneous supplies of high-performance compute (HPC) with competencies around orchestration, routing, and pricing, along with additional proprietary features designed to attract demand and combat margin compression, especially on the inference side. Teams are also beginning to diverge across use cases and GTM, with some focused on incorporating compiler frameworks for efficient inference routing across diverse hardware while others are pioneering distributed model training frameworks atop the compute networks they're building.
We're even starting to see an AI-Fi market emerge with novel economic primitives to turn compute and GPUs into yield-bearing assets or use onchain liquidity to offer data centers an alternative capital source to acquire hardware. The major question here is to what extent DeAI will be developed and deployed on decentralized compute rails or, if as with storage, the gap between ideology and practical needs never sufficiently closes to reach the full potential of the idea.
- Danny
8. Compute Accounting Standards
Relating to networks’ incentivization of decentralized high performance compute, a major challenge in orchestrating heterogeneous compute is the lack of an agreed upon set of standards in accounting for said compute. AI models uniquely add several wrinkles to the output space of HPC, ranging from model variants and quantization to tunable levels of stochasticity via models’ temperature and sampling hyperparameters. Further, AI hardware can introduce more wrinkles via varied outputs based on GPU architectures and versions of CUDA. Ultimately, this results in a need for standards around how models and compute markets tally up their capacities when crossed with heterogenous distributed systems.As a consequence, we’ve seen numerous instances this year across web2 and web3 where models and compute marketplaces have failed to accurately account for the quality and quantity of their compute. This has resulted in users having to audit the true performance of these AI layers by running their own comparative model benchmarks and performing proof-of-work by rate-limiting said compute market.Given the crypto space’s core tenet of verifiability, we hope that the intersection of crypto and AI in 2025 will be more easily verifiable relative to traditional AI. Specifically, it’s important that average users can perform apples-to-apples comparisons on the aspects of a given model or cluster that define its outputs in order to audit and benchmark a system’s performance.
- Aadharsh
9. Probabilistic Privacy Primitives
In “The promise and challenges of crypto + AI applications” Vitalik references a unique challenge in bridging crypto and AI:“In cryptography, open source is the only way to make something truly secure, but in AI, a model (or even its training data) being open greatly increases its vulnerability to adversarial machine learning attacks.”While privacy is not a new research area for blockchains, we believe the proliferation of AI will continue to accelerate the research and usage of the cryptographic primitives that enable privacy. This year has already seen massive strides in privacy-enhancing technologies such as ZK, FHE, TEEs, and MPC for use cases such as private shared state for computing over encrypted data for general applications. Simultaneously, we’ve seen centralized AI giants like Nvidia and Apple using proprietary TEEs for federated learning and private AI inference when holding hardware, firmware, and models constant across systems. With this in mind, we’ll closely follow developments on maintaining privacy for stochastic state transitions as well as how they accelerate the progress of real-world decentralized AI applications on heterogeneous systems––from decentralized private inference to storage/access pipelines for encrypted data and fully sovereign execution environments.
- Aadharsh
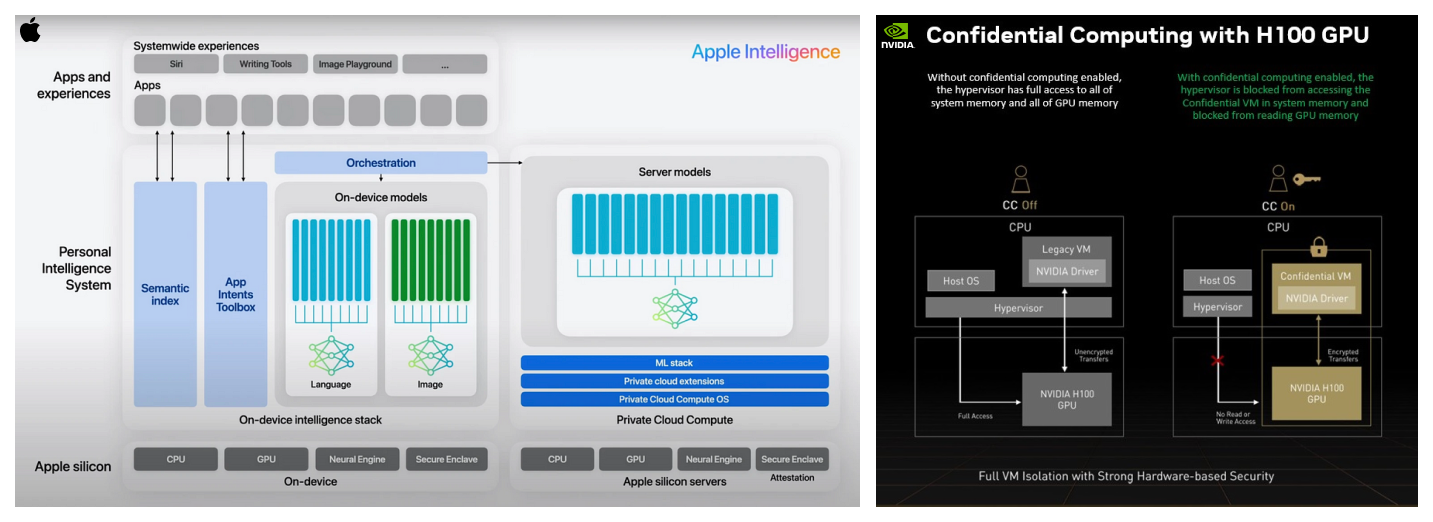
10. Agentic Intents and Next-Gen User Trading Interfaces
One of the most near-term use cases of AI agents is leveraging them to autonomously transact onchain on our behalf. There’s admittedly been a lot of blurry language in the past 12-16 months around what exactly dictates an intent, an agentic action, an agentic intent, solver, agentic solver, etc, and how they differentiate from the more conventional ‘bot’ development from recent years.
Over the next 12 months, we’re excited to see increasingly sophisticated language systems paired with different data types and neural network architectures to advance the overall design space. Will agents transact using the same onchain systems we use today or develop their own tools/methods for transacting onchain? Will LLMs continue to be the backend of these agentic transaction systems or another system entirely? At the interface layer, will users begin transacting using natural language? Will the classic “wallets as browsers” thesis finally come to fruition?
- Danny, Katie, Aadharsh, Dmitriy
—
Disclaimer:
This post is for general information purposes only. It does not constitute investment advice or a recommendation or solicitation to buy or sell any investment and should not be used in the evaluation of the merits of making any investment decision. It should not be relied upon for accounting, legal or tax advice or investment recommendations. You should consult your own advisers as to legal, business, tax, and other related matters concerning any investment or legal matters. Certain information contained in here has been obtained from third-party sources, including from portfolio companies of funds managed by Archetype. This post reflects the current opinions of the authors and is not made on behalf of Archetype or its affiliates and does not necessarily reflect the opinions of Archetype, its affiliates or individuals associated with Archetype. The opinions reflected herein are subject to change without being updated.